Join daily news updates from CleanTechnica on e mail. Or follow us on Google News!
PNNL researchers level towards a wise grid that features machine studying and synthetic intelligence inputs, however with human experience within the loop
Once we flip the sunshine swap in our properties, we have now come to anticipate on the spot entry to electrical energy. Behind the scenes, that reliability is determined by utility operators who’ve developed management programs and fail-safes to maintain the facility flowing.
However occasions are altering quickly, and utility operators face an evolving electrical grid that has turn out to be a fancy community of various vitality sources, rising grid vitality storage choices, and accelerating demand for electrical energy in transportation, computing, and industrial makes use of.
Confronted with the problem of electrical grid modernization, many have known as for supporting utility managers and operators with synthetic intelligence (AI) and machine studying (ML) instruments that may take away a few of their decision-making burden. Understandably, utilities are cautious in adopting new applied sciences when the results of failure are expensive and will have an effect on clients. Moreover, the advantages and enterprise circumstances for these applied sciences usually are not but clear.
Now, a analysis group led by Pacific Northwest Nationwide Laboratory has demystified their rising position within the electrical grid with sensible recommendation. In a comprehensive report, the team points toward a time when ML can become a trusted partner for the nation’s utility operators. As a department of AI, ML makes use of mathematical fashions and real-world knowledge to make choices primarily based on logic and prior information.
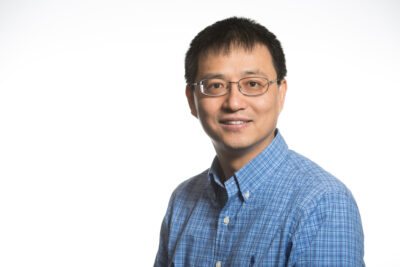
“Electric utility operators are looking for tools that help them understand current system status, to predict what will happen in the future, and then present a recommendation to what kind of actions they need to take to prepare for that future,” stated Yousu Chena PNNL power-system modeling and simulation professional. At present, he leads the Division of Power’s Workplace of Electrical energy Superior Grid Modeling program at PNNL.
Complexity guidelines the electrical grid; machine studying might help us cope
For greater than a century, the nation’s electrical grid operated with centralized vitality manufacturing from coal, gasoline, hydro, and nuclear energy stations. Immediately, that infrastructure is quickly evolving to incorporate a a lot wider number of vitality sources with completely different attributes, alongside a lot larger demand for electrical energy to energy superior manufacturing, transportation, and computing infrastructure.
Trendy knowledge administration and computing methods that embody ML have proven promise to assist handle our energy grid, in keeping with Chen and his colleagues. The most important problem to adoption in 2024 is confidence within the expertise, Chen says.
As outlined within the full report, there are a number of challenges that have to be thoughtfully addressed. They embody:
Reliable solutions. PNNL researchers took a detailed have a look at an ML algorithm utilized to energy programs. After coaching it on actual knowledge from the grid’s Japanese Interconnection, they discovered the algorithm was 85% dependable in its choices. That is known as a “confidence score,” a worth that displays how assured the system is in its choices. When the researchers put human consultants within the loop, they noticed a marked improvement over the system’s assessment of its own decisions. PNNL researchers name the human-in-the-loop rating an “expert-derived confidence,” or EDC rating. They discovered that, on common, when people weighed in on the information, their EDC scores predicted mannequin conduct that the algorithm’s confidence scores couldn’t predict alone.
Cyber threats. Safeguarding data from cyber threats is an ever-present necessity for energy programs, and the usage of machine studying may compound that vulnerability by creating extra potential factors of entry for attackers, until thoughtfully addressed. Nonetheless, anomaly detection algorithms now in improvement at PNNL flag uncommon exercise, comparable to irregular knowledge visitors or irregular knowledge entry patterns, finally enabling faster responses to potential breaches. The PowerDrone project developed AI methods to defend cyber-physical programs, comparable to the facility grid, from cyberattacks.
Mannequin accuracy and flexibility. Computing fashions and digital twin expertise should adapt to altering circumstances. Steady studying and mannequin refinement are vital to keep up effectiveness over time. Chen and his colleagues are creating adaptable fashions that assist predict power-system vulnerability ranges in response to climate and human threats and hazards, whereas additionally proposing potential remediation and restoration methods.
Infrastructure funding and grid modernization. Most energy programs are at the moment not ready to include clever programs. Price and long-term sustainability have to be thought-about rigorously in investing. However as soon as an funding has been made, sensible grids can quickly reply to system adjustments and enhance general effectivity, serving to to recoup an preliminary funding. For instance, PNNL’s Dynamic Contingency Analysis Tool makes use of cascading failure analyses to display for weak spots on the grid, suggesting corrective actions that will be carried out in the course of the response to the occasion. With DCAT, electrical utility corporations can establish energy instability throughout excessive occasions and have a larger probability of stopping a domino impact of energy loss that may result in a blackout.
“We are talking about a fundamental shift in how we operate the grid, moving from one centralized brain, so to speak, to a sponge, adsorbing data from lots of decentralized data sources and providing recommendations based on that data analysis,” stated Chen. “By moving machine learning to local control, instant local decision-making becomes feasible.”
What does that native management seem like?
Demand prediction. By analyzing real-time knowledge, ML might help predict demand to forecast vitality wants extra precisely, serving to stability the grid and scale back waste. Over time, AI may also establish developments in vitality use, enabling higher planning and funding in infrastructure, making our vitality programs extra environment friendly and dependable.
Fault detection and prevention. Sensors put in on gear comparable to transformers, circuit breakers and turbines can constantly monitor working circumstances and feed knowledge to algorithms that predict potential points earlier than they result in system failures. For instance, PNNL’s Shaobu Wang leads a group exploring easy methods to make the grid extra resilient amid unsure climate circumstances. The group is exploring easy methods to use adaptively altering management of wind generators primarily based on real-time operation circumstances utilizing AI approaches to extend reliability and prolong gear lifespan.
Human–machine interplay. Confidence in human–machine interactions is essential for the adoption and acceptance of AI/ML methods within the energy business. Additional analysis might want to deal with defining clear roles for people inside the programs, interfaces, and workflows in order that operators trust within the suggestions made by algorithms.
System reliability. The complexity introduced by renewable integration has led to new grid behaviors and posed challenges to current safety relay settings, which, if not correctly addressed, can probably trigger cascading failures. PNNL’s Xiaoyuan Fan and a group of computational scientists labored intently with the facility business to model preventive controls that stop cascading power failure triggered by intermittent energy inputs.
With fashionable ML and people within the decision-making loop, it will likely be doable to intelligently develop the grid, effectively combine renewable vitality, and considerably harden our infrastructure for a extra strong and dependable nationwide energy system for future generations.
The report of AI/ML expertise in energy programs functions and PNNL analysis actions is supported by the DOE Workplace of Electrical energy by means of the Superior Grid Modeling program. Funding for the reliable AI work was offered by an inner funding at PNN, Pacific Northwest National Laboratory.
By Karyn Hede. Courtesy of PNNL, Department of Energy.
Have a tip for CleanTechnica? Wish to promote? Wish to counsel a visitor for our CleanTech Discuss podcast? Contact us here.
Newest CleanTechnica.TV Movies
CleanTechnica makes use of affiliate hyperlinks. See our coverage here.
CleanTechnica’s Comment Policy